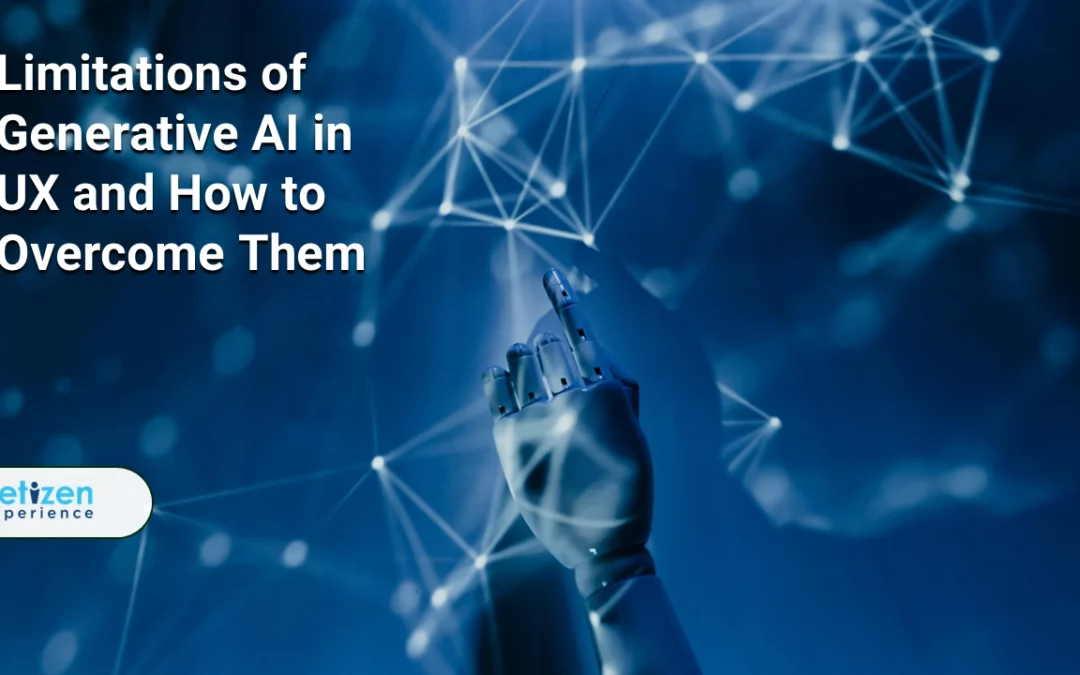
Limitations of Generative AI in UX and How to Overcome Them
Generative AI is transforming UX research, automating tasks like transcribing interviews, analyzing data, and personalizing contents. It’s a big shift from the old, manual ways of working; enabling teams to work more efficiently. But as we embrace AI, we also need to be cautious.
Relying too much on AI can lead to overconfidence in its insights, and we cannot be sure how accurate they are. AI’s training data may also carry biases, raising concerns about fairness. Furthermore, excessive dependence on AI may diminish critical thinking and empathy, which are key to great UX research.
In this Article
Navigating Limitations of Generative AI in UX Research: A Balanced Approach
Given these limitations, understanding how to use AI effectively in UX research is essential. In this article, let’s explore how AI usage fits into each UX research stage and where human expertise is crucial.
Typical UX research phases
Phase 1: Preparation
Defining research objectives under tight deadlines can be tough. AI helps by quickly identifying research gaps, drafting plans, and suggesting participant criteria or recruitment strategies.
However, AI might miss important context or overlook ethical considerations. One way to tackle this is to craft clear input using the CARE structure (Context, Ask, Rules, Examples) and thoroughly review AI-generated outputs.
CARE: Context, Ask, Rules, Examples
(Source: Nngroup.com)
When using AI to create a research plan, providing more details and context using the CARE framework will help generate results that better meet your expectations. If the output still does not meet your needs, refine your prompts by adding missing details or requesting for more options.
Learning to craft effective prompts takes time. It may feel slower at first, but with practice, you will eventually benefit from the outputs of AI.
Clear input, clear output—garbage in, garbage out doesn’t just apply to coding!
Phase 2: Recruitment and Scheduling
Next, let’s look at how AI supports the recruitment and scheduling process, which is typically time-consuming.
AI helps save time by automating outreach, optimizing schedules, and screening candidates. Typically, recruitment tools like User Interviews, Respondent.io, and PlaybookUX use AI algorithms to match your specified criteria (e.g. demographics, job title, device usage, etc.) with participants in their database who fits your study’s needs.
Imagine AI getting all the matching, scheduling and consent done for you – unfortunately, it is not without its limits.
Unfortunately, in this process, AI can also introduce bias and raise privacy concerns depending on its training data.
Take Amazon’s 2018 AI recruitment tool as a reminder of how biased training data led to gender discrimination. The algorithm, trained on biased data, ended up favouring male candidates and penalizing resumes containing the word “women”. Why this happens is because AI can unintentionally “learn” preferences, like favoring men, if for example, it is trained on an unbalanced participant pool.
Hence, the key to using AI effectively is ensuring it stays fair and transparent.
Start by clearly defining your participant criteria — specify who you want to include and what are off-limits.
Next, monitor for biases by reviewing participant selections to ensure they reflect a diverse group. User Interviews has a feature that allows you to opt for manual qualification if you prefer to have more control.
Finally, always prioritize ethics: inform participants what they are agreeing to, obtain consent, and anonymize personal data where possible.
Phase 3: Fieldwork and Testing
Moving to Phase 3, AI can assist in generating interview guides, suggesting follow-up questions, and transcribing sessions. However, one of AI’s known struggles is understanding emotional cues, cultural context, and non-verbal signals.
Consider a scenario in moderated usability testing, where note-taking is key to capturing insights. When a user is confused and says “I don’t get this at all”, an experienced moderator can quickly interpret user emotions, body language, as well as where the user is experiencing the issue within the testing prototype; with the note-taker documenting down these key moments.
In contrast, an AI-powered research tool transcribing the feedback may likely miss the emotional context or the broader interaction.
This gap in contextual note-taking by AI can lead to misinterpretations or cause valuable insights to be missed.
Humans can capture the what, where, and (and why) of user confusion, while AI can only detect simple emotion—without the context behind it.
This is where the human touch becomes essential.
The key is balancing AI’s efficiency with human insights. The days of note-takers stressing over missed insights could become a thing of the past by letting AI handle the note-taking, while you focus on prioritizing noting down users’ subtle emotional cues that AI might miss.
This shift not only ensures that you gather rich and accurate feedback, but also opens up the possibility of reducing manpower. Traditionally, we would have one moderator and one note-taker, but with AI handling the note-taking, one person can effectively manage both roles, leading to greater efficiency.
Phase 4: Analysis and Synthesis of Data
Then, once fieldwork wraps up, the next challenging task is sifting through unstructured data to pull out meaningful insights. AI is a valuable ally here – techniques like sentiment analysis, automated feedback categorization, and predictive analytics enable researchers to swiftly identify patterns and recurring themes, transforming complex data into useful research insights.
However, again, AI is still not perfect.
One notable challenge is that AI-generated findings are often overly general and lack actionable specifics. For example, an AI might conclude that a product “needs improvement”, but fail to specify why users feel this way or to highlight concrete steps for enhancement.
This lack of detail leaves stakeholders without a clear guidance on how to proceed.
Which one of these insights is more helpful?
(Source: Uxcel)
To bridge this gap, it is important to acknowledge that AI may not excel yet in providing practical and actionable guidance.
Instead, the human UX researcher may leverage AI for initial data processing to pinpoint and identify where the UX issues are. Then, use human oversight to interpret the insights within a broader industry and strategic context to translate these findings into actionable insights.
For example, if users struggle to find the “Pay Now” button in your utility app, simply reporting this issue is not enough.
Consider what happened in the session (e.g. visibility issues, lack of color contrast, etc.), factor in best practices, and most importantly, assess the business impact – stakeholders often prioritize understanding the outcome of implementing the proposed recommendations (e.g. increase in on-time bill payments and reduction in late payments).
Phase 5: Communicating and Presenting Findings
Lastly, when turning research into clear, actionable insights, creativity plays a key role. AI is great at organizing findings, generating visuals, and quickly creating presentation templates.
However, AI often falls short in crafting engaging narratives due to its lack of contextual understanding, empathy, and originality.
An AI can assemble a slide deck, but its narratives may lack the personal touch needed to connect with your audience. It’s the human UX researcher that has the real-world work experience to add depth, relevance, and empathy to the story that resonates with your audience.
To maximize the effectiveness of AI, provide clear guidelines on style, reference content, and audience personas. In this stage, it’s about using AI to enhance your work, but let your experience guide the story.
AI Handles the Details, You Bring the Heart: Let AI manage the data, while you add the magic to craft the compelling narrative.
AI as a Partner, Not a Replacement
There is no denying that AI is a powerful tool in UX research, streamlining tasks and revealing patterns we might otherwise overlook. However, it cannot replace human judgment, ethics, or understanding of complex behavior.
Hence, the future of UX research is not AI or humans—it is AI and humans working together.
So tell me, as you work with AI in your research, where do you think it shines, and where do you still find yourself stepping in?
About the Author:
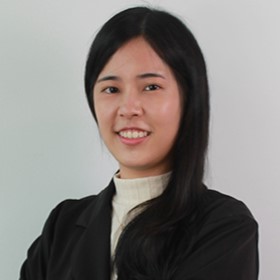
Swan Ling brings nearly three years of UX expertise in user testing, interviews, and research across finance, insurance, and utilities sector as a UX Researcher in Netizen. With a background in psychology and education, she instinctively critiques UX and CX in daily life—when not lost in the outdoors or deep in the forest.